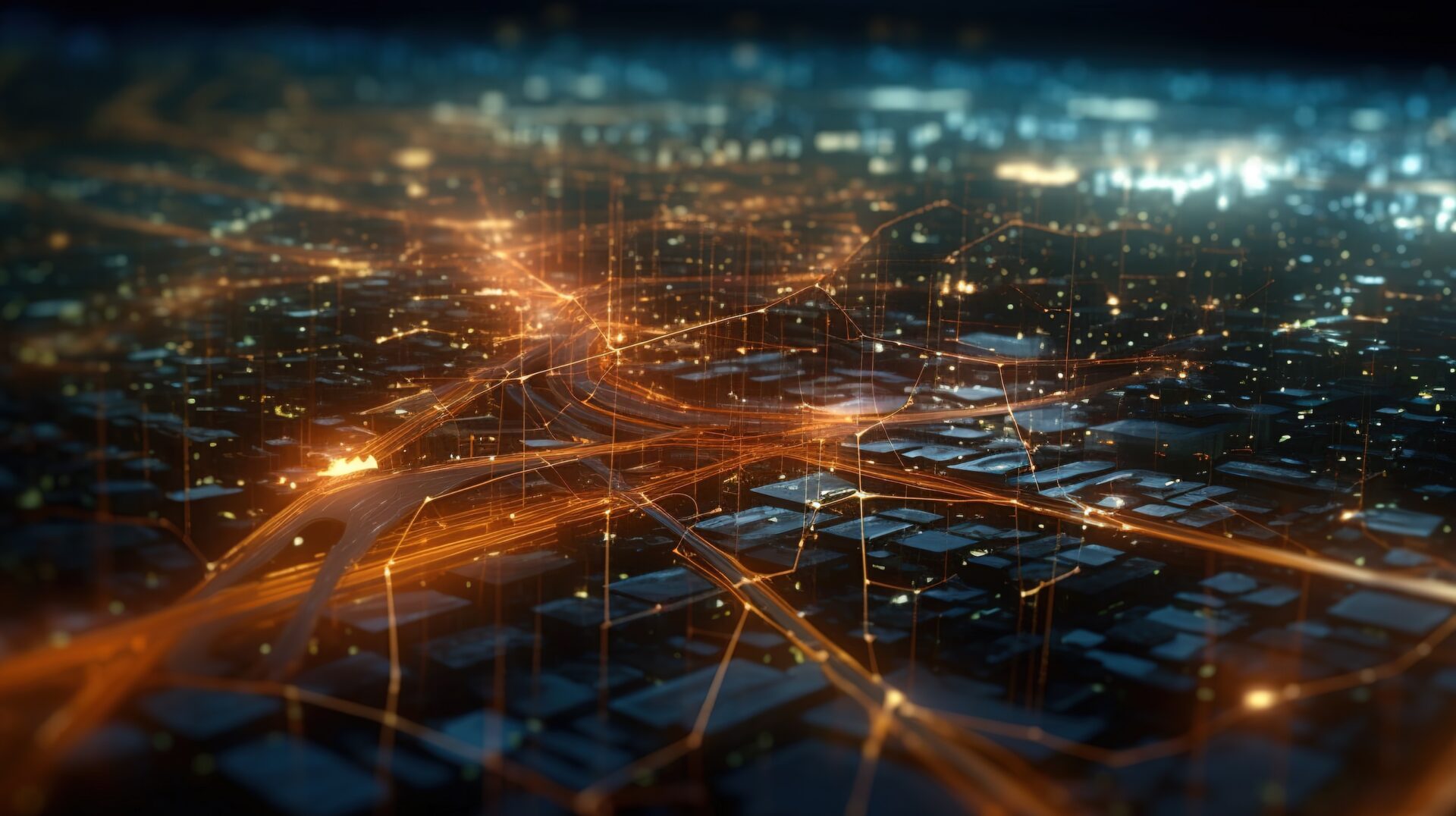
Orange
Industry
Telecommunications
Size
10,000+ employees
Revenue
3,000 MEUR
Project overview
Meet the requirements of new infrastructure inventory reporting regulations with AI and visual data
Orange is one of the players affected by the PCRS, as its infrastructure network covers the whole of France, both above and below ground.
This is why the company called on Alteia’s expertise, particularly in AI and visual data, to enrich its mapping work. By integrating visual data from various sources, in particular high-resolution images from the PCRS, supplemented by other reference systems (Street View, Mapillary, etc.), and using semantic segmentation methods developed by Alteia, Orange is automating the process of identifying and inventorying equipment. This mapping work is based on the Aether analytics solution, the AI platform developed by Alteia, and the scientific and technical expertise of its data science team.
Results
Technical Director
Project highlights
Combining high resolution data, computer vision and GIS for faster and more accurate asset inventory
Image segmentation for object detection
It involves dividing an image into distinct regions or segments based on similarities in color, texture, or other visual attributes. In the context of object detection, image segmentation helps identify and separate individual objects from their background, creating precise boundaries around them.
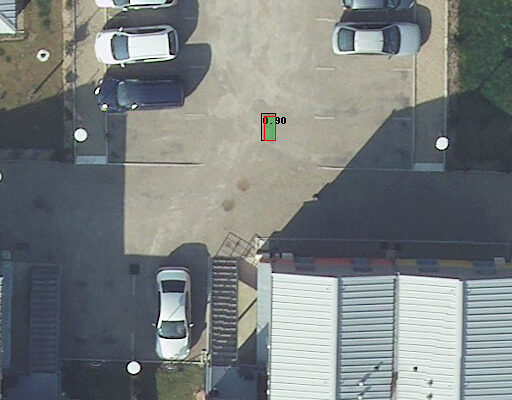
Leveraging Street View data for enhanced accuracy
Combining Street View data with aerial imagery can significantly enhance object detection accuracy. Aerial data offers a global perspective, but it may lack fine-grained details needed for precise object recognition. Google Street View, on the other hand, provides high-resolution, street-level imagery capturing urban and suburban environments in great detail. Integrating these two data sources allows for a multi-modal approach to object detection. By leveraging Street View data, object detection algorithms can benefit from additional context, such as building facades, road signs, and landmarks, improving accuracy, and reducing false positives.
This fusion of data sources is particularly valuable for tasks like urban planning, transportation management, and infrastructure assessment, where a comprehensive understanding of the environment is crucial.
Ultimately, the combination of satellite and Street View data empowers computer vision systems to deliver more reliable and detailed object detection results.

Building detection and GIS synchronization
Using computer vision for GIS (Geographic Information System) database synchronization offers several key advantages.
Firstly, it automates the process of updating and maintaining geographic data, reducing the need for manual data entry and ensuring databases stay current and accurate.
Secondly, computer vision can extract valuable information from imagery and sensors, enhancing the richness and granularity of GIS databases. This results in more detailed and up-to-date spatial information, which is crucial for urban planning, environmental monitoring, and disaster management.
Moreover, computer vision can identify changes and anomalies in geographic data, aiding in the early detection of issues such as land-use changes, infrastructure damage, or natural disasters.
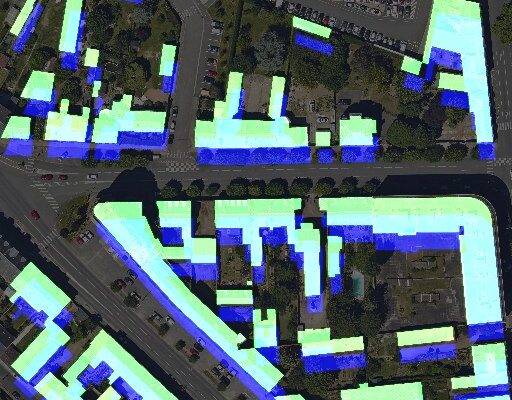
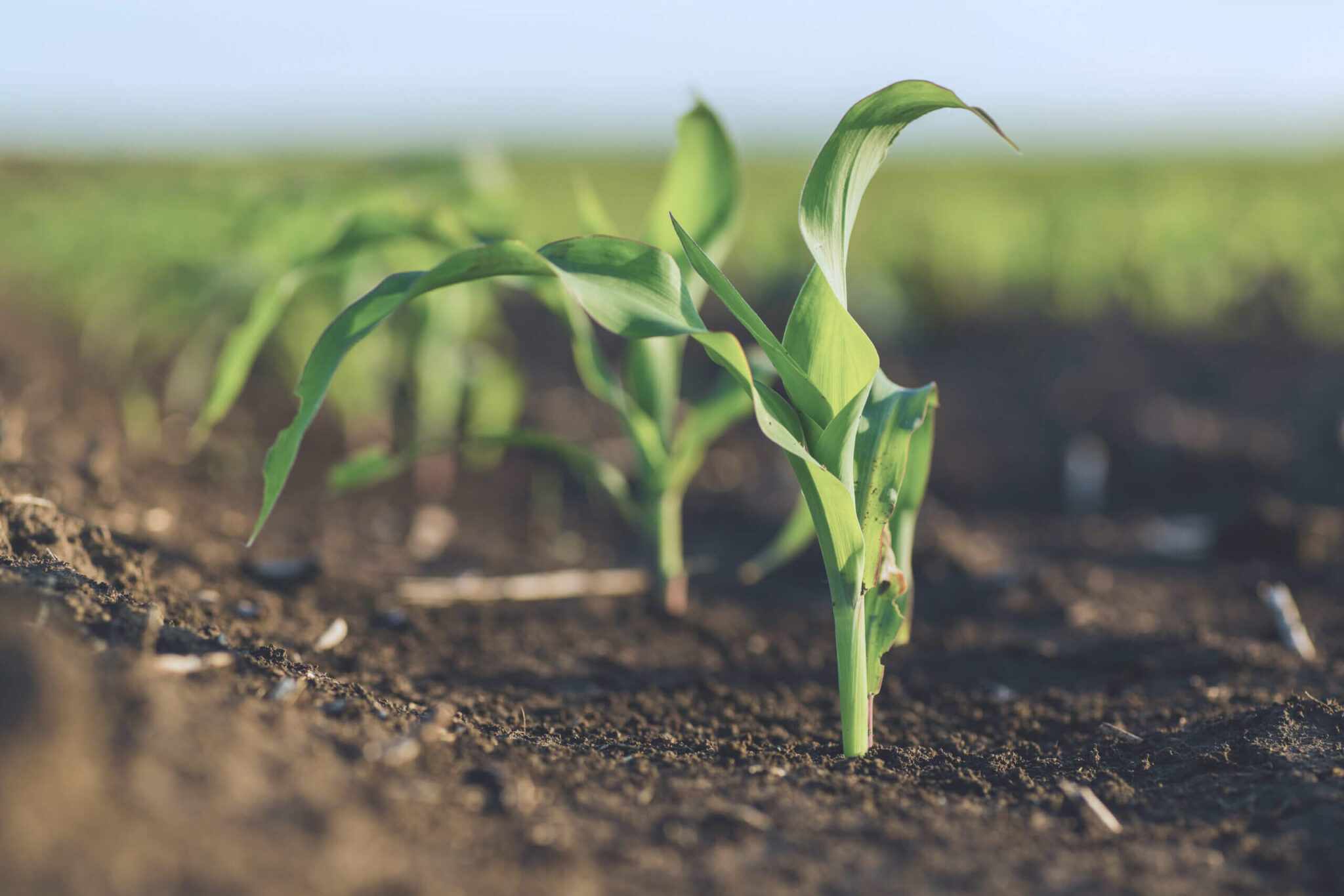