Agriculture & Forestry
Alteia Blog
Three high-value uses of vision AI in agriculture
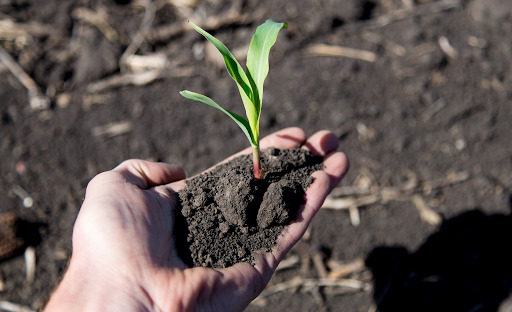
The global agriculture industry is faced with the challenge of feeding a world that demands more food production at a lower cost; to do so, it must adapt fast.
The industry must find ways to grow with fewer chemical inputs, water, land, and labor. And it must do so with less risk to the natural environment in a climate that’s changing erratically.
This article describes three current uses of visual and artificial intelligence in agriculture. Although practical applications of visual AI are relatively new, they already enable these remarkable improvements:
- A company that breeds new crop varieties can now bring them to market 30% faster.
- Farmers who grow crop seeds for commercial use can plan, monitor, and forecast their production with great speed and accuracy, with little effort.
- Manufacturers of farm equipment, crop seeds, and chemicals help farmers reduce production costs, increase yields, and optimize their use of inputs such as seeds, fertilizers, and pesticides.
All three uses already deliver commercial benefits for the Ag suppliers and growers that have deployed them. But, more importantly, visual AI holds great promise for increasing the productivity and sustainability of world agriculture.
1. Seed companies use visual AI to speed the testing of new field crop varieties
Farmers need adaptable crops
A few years ago, one seed company needed 10 years to develop, field-test, and bring a new crop variety to market. How can crop breeders produce adaptable varieties faster with the climate changing fast in many locations?
Companies test new varieties in test plots around the world
Seed companies test new varieties in plots that expose them to varying field conditions: soil types and nutrients, pH, climate, water, and many more. A seed company may test new plant varieties in dozens of locations worldwide. Such experiments reveal where each variety is best suited for commercial use.
Manual monitoring of test plots is time-consuming, expensive, and sometimes inconsistent
The process of monitoring plant growth in test plots is generally slow and labor-intensive. Crop researchers may test one plant variety in dozens of microplots on a single site, and they may operate more than a dozen such test sites in remote locations.
Observers manually record plant and soil conditions in each microplot. They may record data as often as every day throughout a plant’s growth.
The accuracy and consistency of manually collected data tend to vary. The variance may occur because methods are slightly different from one site to another. Likewise, techniques and attention to detail may differ from one observer to the next. And for any one observer, accuracy tends to vary from day to day or even within a day.
Researchers must ask themselves: Are variations in plant data caused by differences in environment, inputs, or variety? Or are they random noise from inconsistent data collection?
Visual AI enables fast, consistent, and cost-effective data collection at scale
Rather than collecting plant-growth data manually, some seed developers now use technology to capture and analyze visual data. For example, they may collect aerial photos from manned or unmanned aircraft. Robots, smartphones, and other devices can also collect useful images.
Cameras or other sensors leverage several technologies to capture different data about crops and fields. For example, they may record both visible and near-infrared wavelengths of light reflected from plants.
The process of data collection is fast. A test site can capture, process, and visualize data for hundreds of microplots within an hour or two. The data can be accurate within a centimeter or less, depending on the technology’s spatial resolution and the distance from the sensor to the ground.
At scale, a global crop breeder can collect data from hundreds or even thousands of microplots worldwide every day. Moreover, they can do so in less time and with less labor than they previously needed to capture data for a single test site.
Visual data monitors plant growth and health
Digital phenotyping is a fast, efficient, accurate, and non-invasive way to evaluate the health of plants. It measures the quality, photosynthetic activity, development, architecture, and growth or biomass productivity of plants.
Visual AI enlists algorithms and indices to monitor plant count, height, health, and density in each microplot. Algorithms can also monitor and measure weed growth.
When questions arise, researchers at a central location can send an alert for a local observer to take a smartphone image of an area in a test plot.
Researchers can also click a mouse to export data for a specific microplot. The exported CSV file may include all the data for a microplot or a group of microplots—including plant count, plant density, plant height, weed count, weed density, etc.
Researchers can load the data into spreadsheets, data visualization tools, or other software for further analysis and reporting.
The ready availability of accurate data can save thousands of hours of labor in a year.
A crop breeder slashes 30% from its development cycle
One crop breeder now uses visual AI at more than 20 test centers around the world. They say their use of the technology has enabled them to cut about 30% from their prior 10-year development cycle.
2. Plant breeders and growers use visual AI to monitor and forecast production of crop seeds
After crop breeders have field-tested new varieties, their next step is to produce seeds in bulk for distribution and sale. Breeders often work with growers who produce crop seeds under contract. In working with growers, breeders have a strong interest in planning, monitoring, and forecasting seed production.
The breeder’s goal is to predict the grower’s yields, not just to record them. Visual AI enables breeders to monitor the growth and health of seed-producing crops. Then growers use algorithms to forecast production for individual farms and aggregate production for all farms.
How production farms are different from test plots
The data-gathering process and technologies are much the same as for genetic testing. But farm fields are physically different from the microplots used in crop testing.
Each farm field has unique boundaries and topography. Farm plots usually lack the clear, rectilinear borders of microplots. Soil and plant conditions within a single field may vary widely from one part of the field to another. Crop rows in a production field may not be straight, depending on the contours of the land. For these and other reasons, the algorithms used for visual AI must be adapted for crop measurement and forecasting.
How visual AI helps forecast seed production
Visual AI algorithms can provide many insights into plant growth. They can:
- Distinguish among crops or plant types.
- Count plants and measure their density, including weeds.
- Infer the growth stage and health of plants by their chlorophyll levels.
By using visual time-series data, AI algorithms can monitor changes in crop conditions. Other algorithms can then correlate crop and field conditions with the eventual seed yields. That’s how growers or breeders can use such data to forecast production volume for a particular field.
Breeders can then add up the forecasts for each field to see a total forecast across all growers. Then, depending on how often growers refresh their data, breeders can have almost immediate updates to their projections. Such updates would be helpful after major weather events such as storms, floods, or droughts.
Visual AI can help forecast production for many other crops
Breeders or growers can adapt visual AI to forecast the production of many kinds of crops. It’s mainly a matter of developing the right algorithms and models for individual crops, then applying the use of visual AI at scale.
Accurate production forecasts can also be useful to crop insurers
Insurers can evaluate crop and field conditions before and after a weather or climate event.
Visual AI algorithms can then quantify the differences between the two. It can objectively assess damages based on any changes in forecasted crop production.
3. Makers of farm equipment and chemicals use visual AI to enable precision agriculture services
Relief for a challenging business
Companies that produce agricultural equipment and chemicals know that it’s hard for farmers to make money consistently. Farmers have long worried about volatile commodity prices, and the number of small, family-owned farms has declined sharply in many countries as the economics of farming has become harder. Unfortunately, the cost of many farming necessities is heading upward: equipment, livestock feed, crop seed, fertilizer, pesticides, fuel, and more.
These days, labor is in short supply. Automation is an obvious solution.
Farms require more significant capital investments. And those investments become riskier as farmers face more severe weather, drought, depletion of water reserves, and long-term changes to the climate.
Manufacturers seek innovation in three areas
Manufacturers who serve farmers are looking for new ways to:
- Make farmers more successful.
- Increase the value of the products they offer farmers.
- Offer farmers data-driven services to make the best use of their products.
- Reduce waste and protect the environment.
Visual AI enables an emerging set of technologies and practices known as precision agriculture or precision farming. Its goals are to increase production and cut farmers’ costs while reducing adverse environmental effects.
Accurate data helps reduce waste
Precision farming reduces waste. It applies technology to optimize inputs such as seed, water, energy, chemicals, labor, and more. Farmers can reduce waste by having access to current, accurate, and granular data about plant and field conditions.
They needn’t plant crops in places where seedlings are unlikely to thrive. Likewise, farmers need not use fertilizers, fungicides, herbicides, and pesticides in areas that don’t need them. With visual data about field and plant conditions, algorithms can analyze where and how much of each input to use.
New farm equipment enables precise control
Digital sensors on new models of farm equipment provide telematic time-series data about GPS location, speed, transmission status, application rates, etc. Then, automated farm equipment can control where and how much seed, water, fertilizer, or other chemicals to apply.
In Summary
These three high-value uses of visual AI are in their early stages of deployment in the agriculture industry. But the technologies are ready for commercial use at scale.
Today, direct investment in visual AI infrastructure may not yet make financial sense for small and mid-sized farmers. Even so, the many benefits of visual AI are already available to progressive farmers of all sizes. That’s thanks to investment, research, and ongoing product development by seed breeders, equipment manufacturers, and producers of Ag chemicals.
For more about the uses of visual AI in agriculture, forestry, and other industries, please visit www.alteia.com. For more information like this, follow Alteia on LinkedIn.
Next articles