Enterprise AI
Alteia Blog
5 Ways Vision AI mitigates climate change through smarter, better industrial practices
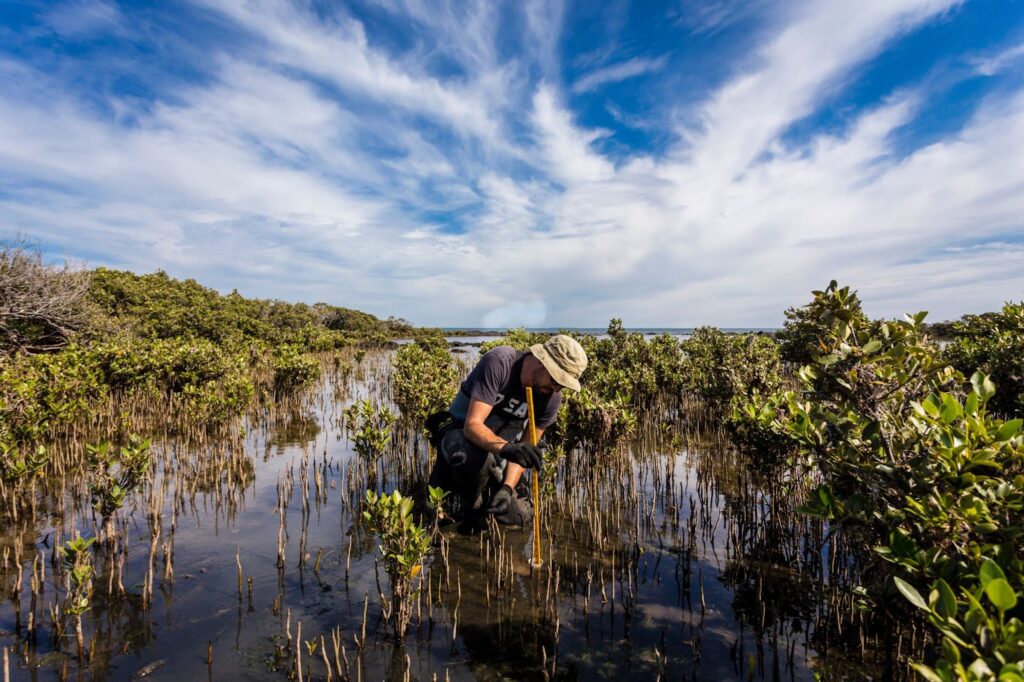
Visual artificial intelligence, or vision AI, provides many tools companies can use to assess or work on their compliance with their ESG goals. Although the technology is relatively new, it is already advanced enough to operate on a very large scale and provide meaningful KPIs.
This article describes industrial applications of vision AI for climate change mitigation, including these:
- Mining
- Agriculture
- Energy
- Forestry
- Waste management
If you’re a decision maker looking for ways to make your company’s operations friendlier to the climate, you’ll read specific examples of how vision AI can help.
The need for immediate climate action
The world must make enormous progress toward mitigating climate change fast. A 2022 United Nations report by the Intergovernment Panel on Climate Change (IPPC) 2022) notes that human-caused emissions of greenhouse gasses have risen almost steadily since 1990. The rate of increase slowed only briefly because of the COVID-19 pandemic, then recovered in 2021 and 2022.
The target is to limit global temperature increase to 1.5°C above pre-industrial levels. That’s the hottest it can get before climate scientists predict the effects of climate change will cascade in ways we may not be able to limit or reverse. The results of such a cascade are hard to predict because natural systems are complex, and the extremes are without precedent in written history.
Climate extremes set many new records around the world in 2021 and 2022. Effects related to rising heat include extreme drought, wildfires, hurricanes, rainstorms, floods, declining populations of plant and animal species, and rising levels of sea water.
As such, EG (Environmental Goals) have become an increasingly crucial aspect of the global agenda, prompting big organizations to take significant steps towards compliance. In today’s rapidly evolving world, the pressing need to combat climate change, protect natural resources, and reduce carbon footprints has placed unprecedented emphasis on sustainability.
Big organizations recognize that aligning with EG is not only essential for mitigating environmental risks but also crucial for maintaining their social license to operate and enhancing their reputation among stakeholders.
These organizations have set ambitious EG targets to minimize their ecological impact. These goals encompass various aspects, including transitioning to renewable energy sources, adopting eco-friendly production practices, implementing waste reduction strategies, and promoting responsible supply chains. Compliance with EG extends beyond internal operations, as organizations are increasingly seeking to influence their partners, suppliers, and even customers to embrace sustainable practices.
To meet these environmental aspirations, big organizations have implemented extensive sustainability frameworks, invested in cutting-edge technologies, and forged strategic partnerships with environmental advocates and governmental bodies. Furthermore, they are making efforts to integrate environmental considerations into their core business strategies, decision-making processes, and long-term planning.
The compliance journey, however, is not without challenges. Big organizations often face hurdles. Data collection and its interpretation is one of them.
Vision AI’s many uses in mitigating climate change
Vision AI offers many tools that organizations can deploy on a massive scale and at reasonable cost to work on their EG or verify compliance.
Organizations can use vision AI to increase efficiency and reduce the costs and risks of their operations.
More efficient practices translate directly into lower carbon emissions, more productive use of land, and the health and preservation of carbon-absorbing forests.
Vision AI can also foster greater resilience to climate change. It does so by enabling better understanding of climate-related events such as flooding, rising sea levels, health of forests and crop lands, and more.
Vision AI also monitors factors that mitigate climate change. It helps organizations comply with regulations, including those related to controlling methane emissions. By helping to control vegetation growth around critical infrastructure such as electrical power lines, it also reduces the risk of wildfires.
How vision AI works
Vision AI is a technology framework that brings together the tools for aggregating, contextualizing, navigating, and extracting the right amount of information to analyze visual data.
By visual data, we mean images and video captured by cameras, laser scanners, and other sensors mounted on aircraft, satellites, ships, and stationary devices.
Combined with powerful software and data processing capabilities, vision AI can make sense of vast amounts of information in multiple formats and from multiple sources.
It can create 2D and 3D digital maps that display multicolored images and overlaid data analysis in real time.
The technology enables organizations to optimize many aspects of their operations. It also collects, processes, analyzes, stores, delivers, and presents visual data so people can use it to make better decisions.
Organizations can use the data in predictive analytics and simulations to model and improve their operations.
5 ways vision AI mitigates climate change
-
Reducing carbon emissions in mines and quarries
The world needs vast quantities of minerals such as copper, nickel, cadmium, and lithium to manufacture batteries that store solar energy and power electric vehicles. But mining is always invasive and environmentally disruptive. It produces large amounts of carbon emissions, mostly from the heavy equipment used to bulldoze, scoop, crush, and transport extracted materials.
The rate of fuel consumption in mining depends on many factors, including the speed at which the trucks are driven, load weight, the slope of the roads, and any aspect of the terrain, such as surface features, debris, or other factors that create resistance for the equipment. Changes to any of these factors affect fuel economy.
Under heavy loads and suboptimal field conditions, a big haul truck like the Caterpillar 777 burns more fuel and can produce as much as about 0.2 tons per hour, i.e. 1.6 tons per 8 hour shift. A mine or quarry that operates a dozen trucks could produce around 20 metric tons of CO2 per shift.
With vision AI, operators can gather precise, site-specific data about individual mine or quarry sites.
Data from vision AI enables operators to model and simulate various haul road configurations, drivers behavior, routes, speeds, deposit points, and fuel consumption. Operators can then choose the model that best reduces fuel use, carbon emissions, and stress on equipment.
Site-specific data enables operators to simulate multiple scenarios with different inputs. They can then implement the scenario that produces the lowest emissions and the lowest cost of diesel fuel.
As an example, the Aether platform by Alteia creates and maintains digital records of mining sites while optimizing haul roads and truck paths. Generated LiDAR point clouds from drone images or laser scanners serve as 3D maps base layers. The Aether platform allows to automatically extract the haul roads information from these maps, allowing to precisely understand haul roads geometry and characteristics. It helps superimpose data coming from the haul trucks onto the maps. It allows to understand areas of improvement and efficiency gains, or run simulations to test alternative routes, or by changing the number of trucks. By doing so, haul roads and truck paths can be optimized resulting in 30% emissions reduction on site.
For more on how vision AI helps optimize mine and quarry operations, see these Alteia blog articles:
- Optimizing Production in Open-Pit Mines: Digital Models and Simulations Using Vision AI as a Data Source
- How the Eramet Group Uses Visual AI to Enable its Digital Transformation
- How Cemex Implements Digital Transformation with Alteia for Quarry Operations Management
-
Speeding crop-seed research and reducing pesticide and herbicide use
Seed researchers and producers continually develop new crop varieties and test their suitability to grow in various environments. Climate change has increased the urgency of their work.
Seed scientists test new crop varieties in outdoor test facilities around the world. The test facilities sow seeds in hundreds or thousands of tiny micro plots, each with various seed varieties and growing conditions.
They then observe the plants and collect information on the performance of each seed variety in each micro plot. The data they collect measures plant growth, density, height, chlorophyll production, and other factors. Researchers combine these data with information about soil conditions, fertilization, moisture, the presence of weeds, and more.
The work is typically time consuming, labor intensive, expensive, and slow. Researchers must collect information from each site and compile it globally. Data collection is often inconsistent because of the lack of resources and small differences in procedure from one test facility to another.
The alternative is to collect data through drones, robots, smartphones, and sensors connected through the internet of things (IoT) .
When growers compile and analyze such data with vision AI, they can monitor the status of plants in thousands of micro plots. Within a few hours, vision AI can count the number of plants, calculate plant-to-soil densities, detect levels of chlorophyll, and distinguish between weeds and crops. It can do so with great accuracy and consistency across sites around the world.
At Alteia, we help agricultural companies integrate artificial intelligence into their daily operations, develop new seed breeding R & D practices, forecast seed production, and help generate more precise weed maps for weed management. For example, the Alteia Field Trial Analysis application is deploying digital phenotyping as a fast, efficient, accurate, and non-invasive way to evaluate the health of plants. As a result, R&D cycles are shortened b 30% and more varieties can be tested.
For more on uses of vision AI in crop research and production, please see these Alteia blog articles:
- Three High-Value Uses of Visual AI in Agriculture
- How Vision AI is building a more sustainable future ?
-
Increasing the resilience of physical infrastructure
Companies in several key industries operate networks of complex physical assets to deliver value to their customers. Such industries include electric utilities, oil and gas, heavy construction, and telecommunications.
Many of the components in their networks are expensive and subject to failure. They require monitoring and maintenance. Climate change has made severe weather more frequent, more extreme, and harder to predict. It has increased the urgency for companies to analyze data about the condition of their assets so they can maintain them in good working order.
Vision AI can collect and monitor large quantities of data about their physical assets fast and cost effectively. From files of video images, it can identify a transmission tower that needs maintenance, or it can spot nearby vegetation that increases risk of a power outage or a wildfire.
Vision AI can provide data that helps organizations map assets, identify potential problems, and even predict which parts of their infrastructure need maintenance. That intelligence helps operators plan, maintain, and even reengineer their networks to be more resilient to climate change.
For instance, Alteia Vision AI’s technology helped a French company that manages 95% of the public electricity distribution network in France to build a smarter network through innovation and technology.
By using a combination of drones and artificial intelligence, Alteia is helping ENEDIS modernize 7,500 kilometers of power lines each year thanks to an eco-friendly smart-grid solution. By replacing only 25% of equipment that require replacement, vision AI is a good way to boost both responsible use of material and efficiency, with a view to optimizing electricity costs for end consumers.
For more about uses of vision AI in asset-intensive industries, please see these articles on Alteia’s blog:
-
Surveying the growth and health of vegetation
Vegetation is a key factor in limiting climate change. Plants remove carbon dioxide from the atmosphere and convert it to oxygen. They also contribute to the hydrologic cycle, which produces humidity, clouds that reflect the sun’s heat, and rain.
The world’s trees sequester carbon more efficiently than any other plants that grow on land. But until recently, owners of forest land could earn more money by cutting trees down than by letting them grow.
Under a nascent system of carbon credits and offsets, forest owners can receive financial incentives to keep their forests intact.
To ensure the fairness and effectiveness of this system, a trusted third party must be able to monitor the health of forests for which the owners receive compensation.
The process of verification and monitoring is complex. Many of the world’s most important tropical rainforests are in remote areas. In such places, it’s often hard to measure the forest-owner’s compliance with the terms of the agreements.
In less remote areas, smaller forests may be widely scattered across big areas. It’s often hard to gather, process, and analyze data efficiently and cost effectively.
Vision AI gathers LiDAR scans and 2D images from aircrafts or drones. It also collects lower-resolution data from satellites. The technology compiles that data and processes it to measure the density and health of forests.
Vision AI algorithms can measure the number, density, and height of trees in an area. They can also distinguish among tree species and infer the health of trees by measuring the presence of chlorophyll in their leaves.
Alteia’s tree counting and advanced tree analysis workflows allow organizations to detect trees in a plantation or forest and measure tree height and canopy. By combining satellite, drone, and ground imagery, our workflow can identify areas with distinct tree signatures, differentiate between vegetation types, and estimate the number of newly planted trees based on detected changes. Our algorithms also provide advanced health and density indicators for specific areas of interest. These parameters allow us to estimate the amount of carbon dioxide these trees will sequester over time.
These capabilities enable carbon markets and regulatory bodies to efficiently verify compliance or noncompliance, even in remote or otherwise inaccessible areas.
For more about using vision AI to monitor tree growth and density, please see this article on Alteia’s website:
-
Detecting and measuring methane emissions
In addition to reducing carbon dioxide in the atmosphere, the world must also cut emissions of methane, a gas created by organic processes. Methane traps atmospheric heat much more powerfully than carbon dioxide. It has 25 times the global-warming potential (GWP) of carbon dioxide over 100 years.
The main sources of methane emissions are oil and gas production, livestock, and waste dumps (from the decomposition of organic materials—mainly food waste).
Detecting and fixing methane emissions worldwide has been identified as a key climate change mitigation strategy, and part of all energy companies ESG goals
Methane can be hard to measure because it diffuses fast. Many collection techniques are unsafe for humans because the gas is explosive.
Aerial and ground observations are becoming an essential part of this strategy, and the need for software solutions that can aggregate, contextualize, and process the heterogeneous data coming from different sensors to generate meaningful reports is key.
In oil and gas production, methane emissions are relatively easy to identify because they are usually associated with physical infrastructure. Pipes and wellheads may leak. And well operators often burn the gas or release it unburned from wells.
At farms and waste-collection facilities, sources of methane are more diverse and harder to pinpoint and contain.
Remote sensors can detect and measure the presence of methane, even in areas where the sources are scattered and inconsistent. Precisely detecting and measuring harmful emissions are crucial steps the world needs to contain and reduce them.
Vision AI can collect and analyze methane data collected from satellites, manned or unmanned aircraft, and stationery sensors. It can map the areas with high methane concentrations, pointing toward remedies and where to apply them.
For example the Aether platform enables companies to import satellite data at a global scale, and leverage short wave infrared (SWIR) images to detect methane plumes from the sky by using Alteia proprietary analytics. Freely accessible data can be leveraged to detect methane point emissions, while higher resolution data can detect small methane plumes.
For more about uses of vision AI to detect and measure methane emissions, please see this Alteia blog article:
Using vision AI to minimize the effects of climate change
With a powerful software platform that can easily be deployed, scaled, and upgraded, vision AI’s role in mitigating climate change is promising.
Vision AI provides visibility, efficiency, and analysis of industrial operations by combining and analyzing vast quantities and types of heterogeneous data. Vision AI enables companies in various industries to turn this data into smart and climate efficient business decisions.
Because mining, agriculture, and energy production are among the industries that most disrupt ecosystems, they also offer the greatest potential gains in mitigating climate change.
With the powerful tools of vision AI, goals crucial for the mitigation of climate change are easier to achieve:
- Detection, measurement, and reduction of greenhouse gas emissions
- Smarter, more efficient uses of land
- Adaptation of food crops to a changing climate
- Reduction in the use of pesticides and chemical fertilizers
- Monitoring and protection of forests and other vegetation
If you’d like to find out more about how you can use vision AI to improve your business operations and meet climate-related challenges, talk to someone at Alteia.
Next articles